Towards better public pandemic policy.
coronavirus·@lifecoma·
0.000 HBDTowards better public pandemic policy.
<center> 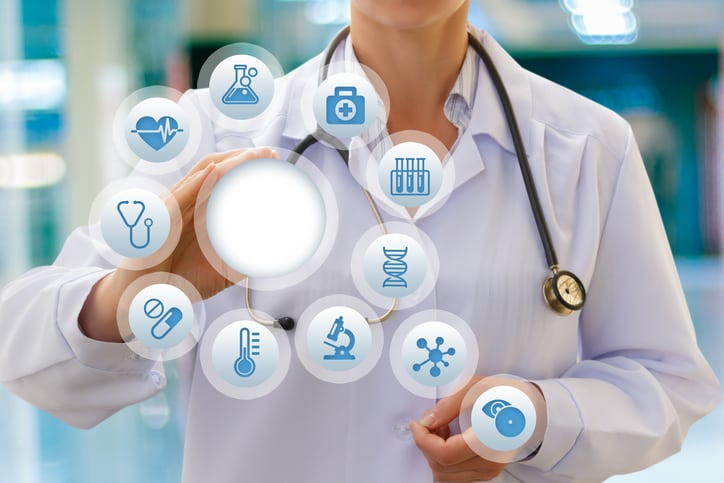 </center> Lots of journalists, and would-be journalists on their social media feeds, are touting half-baked studies of COVID-19 antibody or RNA prevalence as a reason to choose one pandemic policy over another, or worse, as a pretext to spread conspiracy theory junk like, "You've been lied to...". Anybody who cites these studies as a reason to guide public policy, but who can't describe in clear terms how these two types of test differ, and how their sensitivity, specificity, positive predictive value, and negative predictive value, affect their use in various settings, then they actually have nothing of value to say about public policy. Sorry. Having said that, I am sympathetic to the stress we have all been under, and the desire to have some feeling of, if not control, at least understanding of the situation. Grasping at very preliminary information as if it were the new truth is natural. But the pull of jackassery is both strong and subtle in this situation. Please think twice. ##### So here's a tutorial: Sensitivity: the rate at which the test will correctly report someone who is positive. Specificity: the rate at which the test will correctly report someone who is negative. So for the sake of argument, suppose you have a test that is 94% sensitive and 96% specific, which is a good test, and similar to the better COVID-19 antibody tests out there. If you test 100 people who have had COVID-19, you will get 94 true positive tests and 6 false negative tests. If you test 100 people who have never had COVID-19, you will get 96 true negative tests and 4 false positive tests. Every test produces false positives [FP] and false negative [FN] at some rate. What happens when you use this test on a population of 10,000 people, of whom 4% (or 400) have had COVID-19? For the 400 who have had COVID-19, the 94% sensitivity says you will detect 370 true positives. There will be 24 false negatives. For the 9600 who have never been exposed to COVID-19, the 96% sensitivity means that you will correctly report 9216 of them as negative, and incorrectly report 384 of them as positive. There will be a total of 754 positive tests or 7.54% positive in a population where only 4% were truly positive. The positive predictive value [PPV] of a test depends on both the properties inherent to the test, the sensitivity and specificity, but also on the true prevalence of positive individuals in the population. PPV = true positives / total positives, which in this case is: 370 / 754 = 49%, so false positives slightly outnumber true positives. A 49% PPV is not a good test for identifying true positives, but it might still be a good screening test, if confirmatory testing is done later. By itself, a positive test doesn't mean much. The negative predictive value [NPV] also depends on both the properties inherent to the test, and the prevalence of positive individuals. NPV = true negatives / total negatives, which in the case above is: 9216 / (9216 + 24) = 99.74%, so if you test negative, you have a 99.74% chance of being negative in the example population. That is a test you can believe in! You can still use a test like this on a population that is *enriched* in true positives by asking some screening questions, like asking about symptoms of COVID-19, a history of being exposed to known cases of COVID-19, or living in a place where COVID-19 is very prevalent, which is exactly what people doing test like this do. People who are saying "test everyone" are asking for false positives to swamp the true positives in most situations. A test like this can be used as a screening test, which if positive causes a referral to a more specific confirmatory test. I will leave it as an exercise for the reader to compute the PPV and NPV to the example test in a population where 20% of the people are truly positive. I hope this helps.
👍 max.curation, miti, mosquito76, gerber, steemcityrewards, deathwing, emrebeyler, nealmcspadden, daan, exyle, webdeals, themanualbot, someguy123, mys, shitsignals, karja, estream.studios, roleerob, pataty69, heidimarie, enmaart, cadawg, bestboom, freddio, gallerani, raiseup, dlike, triptolemus, determine, permaculturedude, sirbush, vxc, steem.leo, freddio.sport, asteroids, mapxv, leo.syndication, one.life, maxuvd, dappcoder, huaren.news, goodreader, aivote, staryao, accelerator, gerbo, rafalski, fbslo, blockbrothers, nateaguila, samsemilia7, goodcontentbot, fsm-liquid, map10k, triplea.bot, ribary, rycharde, dudiland, bengy, aakom, addax, maxuve, maxuvc, road2horizon, smasssh, tangofever, petrvl, dinamida, belahejna, kryptoformator, jesus.christ, themightyvolcano, ifunnymemes, jacuzzi, ew-and-patterns, rayshiuimages, ralph-rennoldson, pladozero, jeffrey24864, honeybee, amylee, momosan, healthsquared, michaelleeld, maysun, twinkledrop, trendylife, sherrychan, thecatfiles, serenawu, ashely056, helgachen, allanlong, wisemansays, jawnz, brianlam, tonality, picardoh, meepins, liking, tidbits, angelatang, badapple, clappy, poodles, sooty, klobu, lemonhead, fergi, lockhart, hufflepuff, higgs, dobbydoo, aipaws, aristocat, marsbar, denilsonrio, trevorlp97, trevormomo, lifecoma, zafrada, tellmewhy, fefemz, thelittleone, serral, arbitration, tjenkin, babyyoda, spirulina, dooku, dameron, quigon, jabbathehut, normanbates, averill, haldan, fitzroy, mingus, acantha, cerelia, pomeline, holdo, sweetsssj, tagalong,